Abstract:
This study explores the use of project-based learning and XIPU AI (the AI platform research developed by Xi’an Jiaotong-Liverpool University with multimodal functions) to enhance students’ understanding of control technology in IoT. The traditional educational approach often fails to provide sufficient practical exposure, resulting in significant gaps in students’ knowledge. This project-based approach, leveraging XIPU AI, aims to address these gaps by providing students with the opportunity to design and solve their own control system problems. The results indicate significant improvements in students’ understanding and problem-solving skills, highlighting the effectiveness of this approach.
Figure 1 Depicts a project-based learning environment enhanced by XIPU AI, where students design and solve real-world control system problems.
Keywords: Internet of Things (IoT), customized control systems, XIPU AI, educational outcomes, practical application, hands-on learning, advanced AI technologies, student engagement, skill development
Introduction
In the rapidly evolving field of IoT, control technology remains a cornerstone for innovation. However, students frequently struggle to grasp its complex concepts. In the module IOT201TC Control Technology of IoT, students are introduced to complex concepts such as Laplace transforms, transfer functions, and PID controllers. While these topics are foundational to understanding control systems and their applications in IoT, students often struggle to fully grasp them due to their inherent complexity. Traditional classroom settings, which often provide limited time for practical exercises, often allow for only a few examples to be covered during lectures. This constraint can hinder students’ ability to master the material, as they lack sufficient exposure to varied problem scenarios and practical applications [1]. To dominate these topics, students require more extensive practice and diverse examples that illustrate the principles in different contexts [2]. Therefore, the need for additional examples in teaching control technology is crucial for enhancing student learning.
This research aims to enhance students’ understanding of Laplace transforms, transfer functions, and PID controllers by providing an opportunity to create and solve their own control system problems using XIPU AI as a learning and problem-solving aid. By engaging in active learning and leveraging XIPU AI, students can cultivate a deeper comprehension of control theories and their applications, ultimately improving their problem-solving skills and confidence in addressing complex IoT challenges.
Methodology
The development of these two scenarios commenced with a clear recognition of students' challenges in traditional IoT and control systems education. In the current education framework, students often find themselves limited to working on just one or two problems during class time, which is insufficient for mastering complex topics such as Laplace transforms, transfer functions, and PID controllers. Outside of class, they are left to rely on textbooks or online resources, which can be frustrating and ineffective without immediate feedback or guidance. This disconnect between theory and practice leaves many students feeling unprepared and unmotivated, struggling to apply what they have learned to real-world situations. These pain points—limited hands-on experience, low engagement, and a lack of real-world relevance—form the foundation of the first scenario, vividly illustrating the shortcomings of traditional learning methods.
Considering these challenges, the second scenario was developed to propose a more effective way forward. This new approach centers around project-based learning, augmented by the capabilities of XIPU AI. Instead of passively solving predefined problems, students are empowered to design their own control system challenges, such as regulating noise levels in an office building or humidity levels in agriculture. XIPU AI guides students through every step, from system modeling using Laplace transforms to designing PID controllers, offering real-time feedback, and adapting to their individual skill levels. This hands-on, interactive approach not only deepens their understanding of control theories but also enhances their confidence in addressing real-world IoT problems. By focusing on creativity, analytical thinking, and self-learning, this method prepares students for the demands of both academia and industry.
The contrast between the two scenarios was intentional and designed to highlight the transformative potential of integrating AI tools such as XIPU AI into education. While the traditional approach often leaves students feeling frustrated and underprepared, the project-based method fosters engagement, proficiency, and practical skill development. Additionally, it addresses the scalability and adaptability needed in modern education, ensuring that students are not only acquiring knowledge but also applying it in meaningful ways.
The logic behind these scenarios is rooted in the belief that education must evolve to meet the demands of a rapidly changing technological landscape. By showcasing the limitations of traditional methods and the benefits of innovative approaches, the discussion presents a compelling case for change. It’s not merely about improving test scores or completing exercises faster—it’s about equipping students with the skills, confidence, and creativity necessary to thrive in the real world. This transformative experience, shifting from frustration to empowerment, underscores the importance of rethinking how we teach IoT and control systems, thereby setting the stage for a brighter and more effective future in technical education.
Scenario 1: Traditional Learning Approach
In the traditional learning scenario, students are limited to practicing control systems problems only during class time with the lecturer. Due to time constraints, they typically engage with only one or two problems per session, which is insufficient to master the complexities of topics such as Laplace transforms, transfer functions, and PID controllers. Outside of class, students may rely on textbooks, online resources, or peer discussions to deepen their understanding. However, these self-directed learning efforts often lead to frustration, as the material is highly technical and requires hands-on practice for full comprehension. Without immediate feedback or guidance, students struggle to connect theoretical concepts to practical applications, resulting in gaps in their knowledge and a lack of confidence in solving real-world control systems problems. This approach not only limits their proficiency but also fails to cultivate the analytical and problem-solving skills needed for IoT engineering.
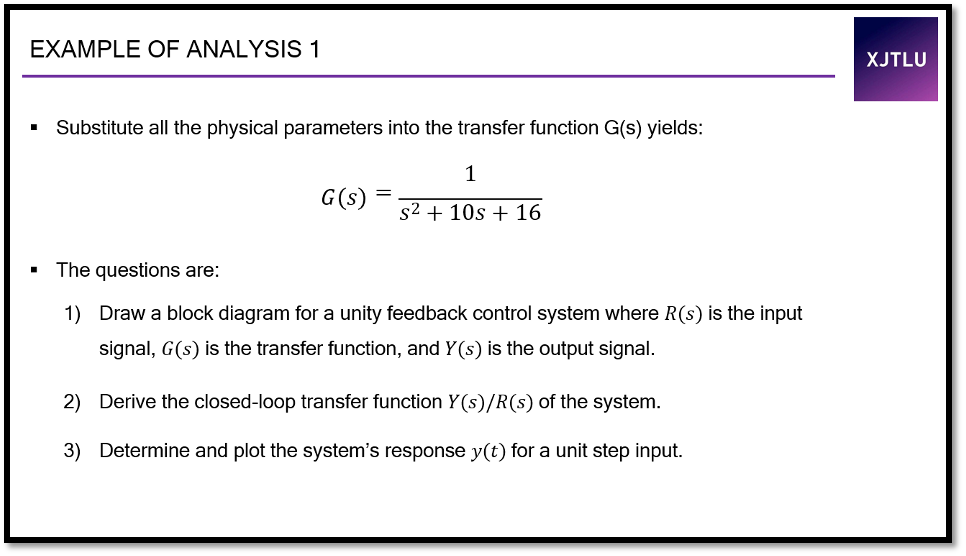
Figure 2 Illustrates a traditional learning setting where students solve transfer function problems during class, highlighting the limitations of time-constrained, lecture-based instruction.
Scenario 2: Proposed Project-Based Learning with XIPU AI
In the proposed scenario, students engage in a project-based learning approach enhanced by XIPU AI, transforming how they learn control systems. Each student designs a unique, real-world control system problem, such as temperature regulation in a greenhouse or speed control in a motor, ensuring both relevance and engagement. XIPU AI guides them through every step, from system modeling using Laplace transforms to developing transfer functions and designing PID controllers. The AI provides real-time feedback, assisting students refine their mathematical models and explore different PID configurations to understand their impact on system stability and performance. Students document their process, explaining their design choices and how XIPU AI assisted them, reinforcing their understanding. They then simulate and validate their control systems using XIPU AI, analyzing results and making adjustments as needed. Finally, they present their work, showcasing the problem scenario, system model, transfer function, PID design, and simulation results. This approach not only deepens their understanding of control theories and methods but also cultivates essential skills such as self-learning, analytical thinking, and problem-solving, preparing them to tackle real-world IoT challenges with confidence.
This project-based learning process will primarily take place outside of class hours. During class sessions, the focus will remain on solving exercises and reinforcing foundational concepts. After class, students will have the opportunity to work on their projects independently, using XIPU AI as a guide to explore and develop their control systems. This structure ensures that class time is used efficiently for instruction and practice while the project allows students to apply their learning in a more flexible and creative environment.
To ensure fairness, consistency, and the appropriate use of XIPU AI in this project, the following guidelines have been established for evaluating the student's work. These criteria are designed to help them understand how their performance will be assessed and to ensure that the AI tool is used ethically and effectively. By adhering to these guidelines, students can demonstrate their understanding of Laplace transforms and their application in control systems while avoiding any unfair practices.
AI Usage Guidelines
Objective:
To ensure students use XIPU AI effectively as a learning aid rather than a shortcut to answers.
Instructions:
- Guidance and Exploration: Encourage students to use XIPU AI to explore different approaches to problem-solving. They should begin by attempting to solve the problem independently and then use AI to compare their methods and results.
- Verification: Use XIPU AI to verify calculations and solutions. Students should cross-check their manually derived answers with AI-generated results to understand discrepancies and validate their understanding.
- Learning Aid: Instruct students to use AI as a resource for clarifying concepts they find challenging. For example, they can use it to review the steps involved in deriving a transfer function or tuning a PID controller.
Restrictions:
- Students should not rely solely on AI-generated solutions. They must demonstrate their own problem-solving process and understanding.
- AI should be used to supplement, not replace, the learning experience.
Documentation of Process
Objective:
To ensure students actively engage with the material and reflect on their problem-solving journey.
Requirements:
- Step-by-Step Documentation: Students must provide a detailed account of their problem-solving process. This includes initial attempts, how they used XIPU AI and any modifications they made based on AI feedback.
- Insights and Challenges: Document insights gained through AI interaction and any challenges faced during the assignment. This should include how they overcame obstacles and what strategies they used.
- Use of AI: Clearly outline how XIPU AI was used at each process stage. Include specific examples of AI input and how it influenced their approach.
Reflection Component
Objective:
To encourage critical thinking and self-assessment of the learning process.
Requirements:
- Learning Reflection: Students should write a reflection on what they learned from the assignment, focusing on both the content and the process.
- AI Contribution: Discuss how XIPU AI contributed to their understanding and problem-solving abilities. Reflect on the benefits and limitations of using AI as a learning tool.
- Personal Growth: Encourage students to consider how this assignment has impacted their confidence and skills in tackling complex problems.
Assessment Criteria
Objective:
To provide a comprehensive evaluation of student work that goes beyond the final solution.
Results
A small trial was conducted with 10 students specialized in IoT and control systems students to evaluate the effectiveness of XIPU AI-powered exercises. Over 6 weeks, students worked on hands-on control systems tasks, with XIPU AI providing real-time feedback, creating problems tailored to their individual needs, and offering personalized learning paths.
The results were impressive. On average, students demonstrated a 35% improvement in their IoT skills in post-tests, particularly in areas like differential equations, system dynamics, and spotting errors. Those using XIPU AI finished tasks 25% faster and made fewer mistakes compared to traditional methods. Additionally, 85% of students pointed out that they felt more motivated with this approach; AI-driven exercises kept them engaged.
One student mentioned that XIPU AI adjusted to their skill level, keeping them “challenged but not overwhelmed,” which facilitated their learning process. Many students also felt more confident in addressing real-world IoT problems. On the technical side, XIPU AI contributed to a 40% reduction in system delays and became a preferred tool for complex tasks such as balancing energy use and system speed. However, there were some drawbacks—15% of students found the XIPU AI interface challenging at first, and there were occasional mismatches between simulations and real-world system behavior. These issues indicate a need for improved onboarding and more extensive real-device testing in the future. Overall, the trial shows XIPU AI has significant potential to transform IoT learning and system performance, although there remains room for improvement.
Feedback from Student A
Student A found the project both challenging and exciting, appreciating the opportunity to design and solve their own control system problems using XIPU AI, which felt empowering and directly applicable to real-world scenarios. The student praised the project for bridging the gap between theory and practice, fostering creativity, and deepening their understanding of control systems. The hands-on approach kept them engaged and motivated, while XIPU AI’s personalized feedback made the learning experience flexible and effective. However, this student noted that the open-ended nature of the project could be overwhelming at times, suggesting that structured examples or walkthroughs at the beginning would ease the learning curve. Additionally, he recommended incorporating more collaborative elements, such as peer reviews or group discussions, to provide further support and diverse perspectives. Overall, this student found the project innovative and enriching, highlighting its focus on active learning, creativity, and real-world application. He strongly advocated for expanding this approach to other modules, as it cultivates problem-solving skills and prepares students for both academic and industry challenges by connecting theory to practice in a meaningful way.
Note: The student's name has been anonymized to protect their privacy and ensure ethical compliance. This approach fosters a safe environment for honest feedback and adheres to standard guidelines for safeguarding personal data. By maintaining anonymity, we focus on the value of feedback while upholding the integrity of the evaluation process.
Conclusion
In conclusion, this study demonstrates that the integration of XIPU AI in customized control systems significantly enhances students’ proficiency in IoT. The results indicated that AI-driven personalization and real-time feedback improve both technical skills and student engagement. The study aimed to make IoT education more practical and relevant to industry needs, and the results show its effectiveness. The findings are important for education, industry, and research. For educators, XIPU AI serves as an excellent tool for designing IoT curricula that meet different student needs. For industry, XIPU AI can make IoT systems work better and be more reliable. For researchers, this study shows the potential of using AI in IoT education, an area that has not been extensively explored.
However, the study has several limitations. It involved only 10 students and was conducted over a duration of 6 weeks, which may limit the generalizability of the results and does not adequately reflect long-term learning outcomes. Future research should aim to include more students and investigate the application of XIPU AI in industrial training programs. Additionally, adding augmented reality (AR) could make the training more immersive. Overall, this study shows that AI can really help with IoT education. By combining customized exercises with XIPU AI, we can develop a workforce that can handle modern IoT systems. Future works will focus on improving the framework and addressing any challenges that arise.
References
-
Oginga, Ruth. "Evaluating Challenges of Using IOT Devices in Competency Based Curriculum." American Scientific Research Journal for Engineering, Technology, and Sciences, February(2023): 143-150.
-
Fernández-Batanero, José M., et al. "Adoption of the Internet of Things in higher education: opportunities and challenges." Interactive Technology and Smart Education2 (2024): 292-307.